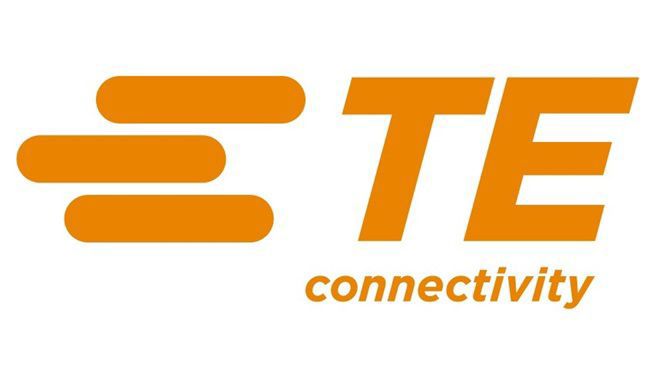
TE Connectivity is a $14B designer and manufacturer of connectivity and sensor products for the automotive, industrial, data communications, aerospace, defense, medical, petroleum, consumer, and energy sectors. Several years ago, TE invested in a major digital transformation which included a new digital platform, ecommerce capabilities, product information management (PIM), and product taxonomy. The new product taxonomy was developed to migrate product data from the existing product database to the new PIM. This was a massive exercise across 18 product divisions, over 700 unique categories of products, and hundreds of thousands of products.
Because the data migration had to move quickly to keep pace with the launch of the new website, the taxonomy and product data teams didn’t have time to standardize any attributes that were shared across large numbers of products (like “color” or “voltage”), nor to normalize data values. Instead, each category had its own local version of attributes. This means if 100 categories needed a “voltage” attribute, 100 unique attributes were created. As a result, TE’s product taxonomy was massive and complex, difficult to govern and manage, and product data quality was not optimized.
As an antidote to this problem, TE recently adopted a “Preferred Attribute” approach for governing product data. Attributes like “color” and “voltage” were standardized (defined in a single place) so they could be used globally across the taxonomy. So now instead of 100 “voltage” attributes in 100 categories, there was just one, and it was used in 100 categories.
Likewise, TE standardized lists of values (“LOVs”) for enumerated attributes. LOVs are the sorts of things you see in drop-down lists. For example, in the case of the “color” attribute, it might have a list of values that looks like “Red | Orange | Yellow | Green | Blue | Indigo | Violet | Black | White | Gray”.
TE engaged Dakota Systems to standardize product data attributes. Dakota’s tasks would be to map the legacy category-specific attributes to the Preferred Attributes and recommend additions to the Preferred Attribute list. TE and Dakota collaborated on a work process and deliverables formats to meet TE’s requirements.
Dakota used attribute prevalence and fill rates to systematically determine the proposed disposition of every attribute in the product catalog. Dakota recommended whether they should be:
-
mapped to existing Preferred Attributes (because they were the same concept),
-
become new Preferred Attributes (because they were a unique concept not matching an existing Preferred Attribute),
-
or go away (because they were not widely used, not observed in the marketplace, and/or not filled with data values on most products).
After Dakota mapped attributes to the Preferred list, the TE team systematically normalized the attribute values for all the product data in the category. This means that they will all have the same unit of measurement, the same decimal precision, and would use the standard LOV entries.
Because of the scale of the standardization project, TE split the project into phases over several years. The Dakota team completed attribute standardization working independently, one category at a time, passing entire categories back to TE when they were completed. The TE team worked through the categories progressively, processing the proposed Preferred Attributes through their taxonomy governance process, then normalizing the data.
Standardizing attributes to a preferred attribute list and preferred LOVs improved the efficiency of product data operations:
-
Data Governance is easier. Standardizing to a set of preferred attributes makes managing the taxonomy easier because there are simply fewer attributes to manage. Having fewer attributes makes it is less likely that you will create a redundant attribute by mistake. The typical level 1 product category in this project had a 50% decrease in the total number of attributes with standardization.
-
Data fill rates go up. In many cases, categories had multiple attributes for the same concept, with the value filled for only one or the other on most products. This may have occurred when similar product categories from different divisions were merged or a company was acquired with similar products, and both legacy attributes were preserved. When redundant attributes are mapped to Preferred Attribute, the fill rate goes up (approaching 100%) and the number of attributes goes down (by N-1). In this project, the fill rate for the largest category went from 19% to 45% with 50% standardization, and up to 66% when standardization was complete.
-
Product data quality is better. Standardizing LOV entries reduces the total number of options presented to product data specialists who are onboarding products because you consolidate values with the same meaning to a single LOV entry. For example, a “plating material” attribute could be copper applied over a nickel base. Without standardization, the LOVs might include “Copper over nickel | Copper-nickel | Copper-nickle | Cu-Ni | Cupro-nickel | Copper/nickel | Copper + Nickel” and so on.
-
Time to onboard a product goes down. Fewer attributes require less data entry time. If the number of attributes goes down by 20% the time to complete data entry goes down proportionally.
-
Product teams onboard more products. Assuming Business Units invest the same amount of time for product on-board and data enrichment, they can onboard 20% more products in the same amount of time. This helps reduce the backlog of products waiting in queue to be merchandised on the web. In fact, for the largest category in this project, the number of products online increased by 25% with half of the attributes standardized and by a total of 45% when standardization and normalization were complete.
Standardizing attributes and normalizing their values improved the performance and usability of product pages in the online catalog:
-
Product catalog page views increase. For the first large category that Dakota normalized – Printed Circuit Board Connectors – organic findability for products (in terms of total pageviews) increased 20% after standardization and normalization. Pageviews increased 4% after the first 50% of attributes were standardized and normalized, and another 16% when standardization was complete.
-
Search and compare filters are more effective. Standardizing LOVs makes search filters and product compare screens work better because you consolidate values with the same meaning to a single LOV entry as illustrated above with contact plating materials. Without standardization, website visitors can’t filter a list down to similar products without selecting each variation.
-
Product compare is more usable. Product compare screens work better because redundant attributes are consolidated and their values are normalized. This is particularly true for LOV attributes and ranges.
The standardization and normalization project was a true collaboration between Dakota and TE. The project enabled the TE team to accelerate their efforts to increase data quality, data fill rates, and the percentage of products represented in the online product catalog. With better quality data, TE’s product pages perform better, resulting in increased sales online and through their distributor and direct channels.